Hannah Lawrence
I am a PhD student in machine learning at MIT, where I am fortunate to be advised by Ankur Moitra. I am also a member of the wonderful Atomic Architects, led by Tess Smidt. Previously, I was a summer research intern at the Open Catalyst Team at Meta FAIR, studying equivariance for chemistry applications.
Before graduate school, I was a research analyst at the Center for Computational Mathematics of the Flatiron Institute in New York, where I worked on developing algorithms at the interface of equivariant deep learning and signal processing for cryoEM. Broadly, I enjoy developing theoretically principled tools for deep learning (often in scientific or image domains), with a focus on learning with symmetries.
I spent summer 2019 at Microsoft Research, where I was lucky to be mentored by Cameron Musco. I've also spent productive summers at Reservoir Labs and the Center for Computational Biology. I was an undergrad at Yale in applied math and computer science, where I had the good fortune of being advised by Amin Karbasi and Dan Spielman.
Finally, I co-founded the Boston Symmetry Group, which hosts a recurring workshop for researchers interested in symmetries in machine learning. Follow us on Twitter, shoot us an email, or join our mailing list if you're interested in attending!
Email  / 
Github  / 
LinkedIn  / 
Twitter  / 
Google Scholar   
|
|
Research
My primary research interests include symmetry-aware (equivariant) machine learning and scientific applications. In addition, I enjoy developing theoretically principled tools for deep learning, for applications from vision to interpretability to PDEs.
Here is a non-exhaustive list of a few high-level questions I've been thinking about recently (or at least, the last time I updated this website):
- What kinds of approximate symmetries arise in practice, e.g. in scientific applications? How should this structure inform our choice of architecture, and when is approximate symmetry still a powerful enough inductive bias to benefit learning?
- What is the role of equivariance, e.g. to permutations, in large language models (LLMs)? To what extent is equivariance learned? To what extent should it be enforced?
- How can we harness equivariance to learn useful representations, especially in applications with complicated symmetries (such as PDEs)?
- How can we make canonicalization work, in theory and in practice, as an approach for enforcing symmetries?
- Does equivariance have a role to play in NLP? In fairness?
|
|
Artificial Intelligence for Science in
Quantum, Atomistic, and Continuum
Systems
Xuan Zhang*,
Limei Wang*,
Jacob Helwig*,
Youzhi Luo*,
Cong Fu*,
Yaochen Xie*,
...,
Hannah Lawrence,
...,
Shuiwang Ji
Under review, 2023.
A survey of machine learning for physics.
|
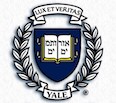 |
Organizer, Boston Symmetry Day, Fall and Spring 2023
Teaching Assistant, 6.S966 Symmetry and its Applications to Machine Learning, Spring 2023
Hertz Foundation Summer Workshop Committee, Fall 2021 and Spring 2022
Women in Learning Theory Mentor, Spring 2020
Applied Math Departmental Student Advisory Committee, Spring 2019
Dean's Committee on Science and Quantitative Reasoning, Fall 2018
Undergraduate Learning Assistant, CS 365 (Design and Analysis of Algorithms), Spring 2018
Undergraduate Learning Assistant, CS 223 (Data Structures and Algorithms), Spring 2017
Undergraduate Learning Assistant, CS 201 (Introduction to Computer Science), Fall 2017
|
|